In medical research there is a growing amount of data. It can therefore benefit from the possibilities and innovations that data science brings to the table. Whether it is analysing complex datasets to uncover new information or automating research outcomes, data science is able to help and deliver value.
Our project for Stichting Hartekind
Each year 1.500 children are born with a congenital heart disease in the Netherlands. About two-thirds of these children needs a major open heart surgery or heart catheterization. Fifty years ago 75% of these children died due to their heart disease. Nowadays 150 (10%) do not survive. Of course this is still 150 too many! Stichting Hartekind carries out research in order to decrease this number even further. It is the only charity who does this exclusively for pediatric cardiology.
During our Collective week 2019 we helped Stichting Hartekind with their ongoing research on pediatric cardiology. By providing new insights and tools we are able to help them with further research. We focused on four projects, predominantly concerning cardiopulmonary exercise tests (CPET), i.e. endurance tests. For these tests children perform an exercise on a bike. During the tests researchers and doctors measure various performance indicators while they increase the resistance of the bike until the child reaches maximum endurance. With these tests doctors are able to assess whether the child needs special attention or even surgery. Our research topics ranged from clustering patients and biomarkers, to predicting clinical events based on the outcomes of the endurance tests, to automating the detection of the anaerobic threshold.
Of course, we were eager to demonstrate how data science can help medical research. Within four days we could make a valuable impact on each of the four research topics. This demonstrates that it is possible to develop solutions efficiently in a wide range of topics. Furthermore, we show that the innovations and possibilities that data science brings to the table can be used in different corners of research.
Examples of how data science can help medical research
Utilizing advanced data science techniques: clustering CPET outcomes
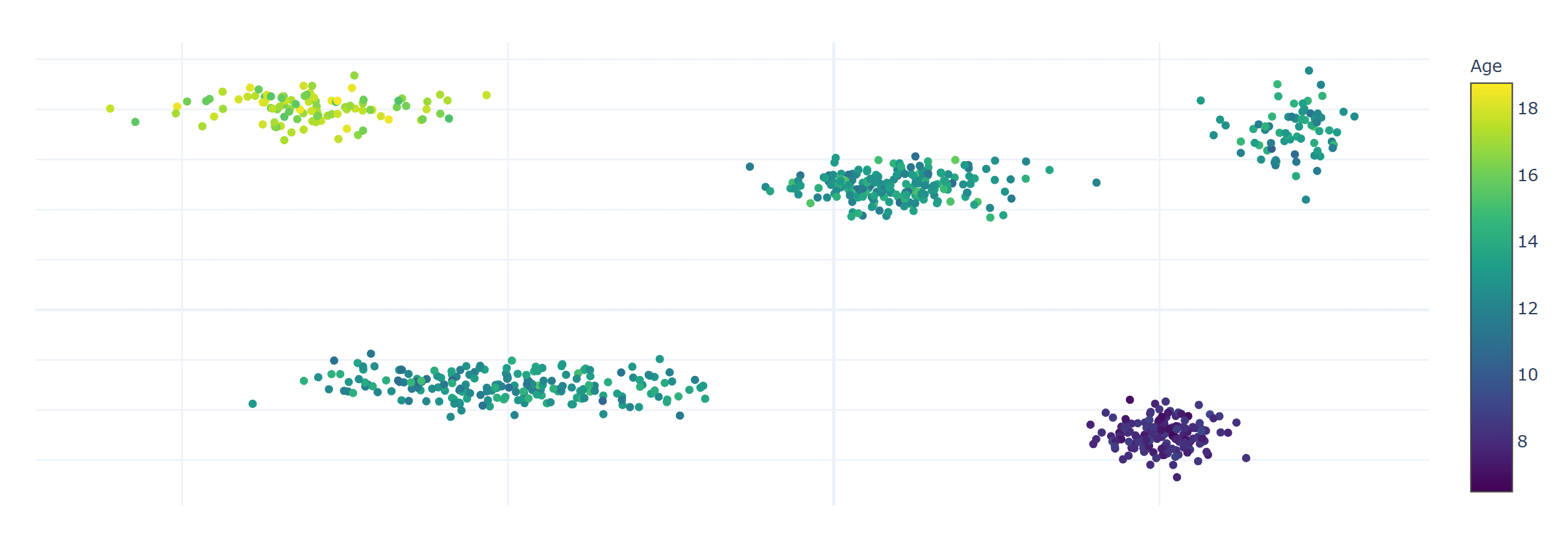
Figure 1. Clustering patients with an unsupervised learning model. (dummy data)
Within the field of data science various methods are available for analysing large and complex datasets. Stichting Hartekind asked us to find clusters of patients based solely on the outcome of their cardiopulmonary exercise test (CPET). Researchers can then map patient attributes such as disease, age and weight to these clusters. By doing so, we hope to understand which patient groups are comparable and use these as benchmarks for future CPET outcomes. The dataset itself consisted of thousands of tests, each containing numerous performance measurements. By using an unsupervised learning model we detected a number of clusters within the data. With the aid of dimensionality reduction techniques, we visualized these clusters and created a convenient dashboard (see figure 1.) to understand which characteristics were clustered together. Further research is done to analyse each cluster and provide medical interpretation of the results.
Improving predictions by using more information
Figure 2. CPET results are input for our prediction model. The model takes all information into account, also from the recovery phase. (dummy data)
Stichting Hartekind also asked us if we could predict clinical events based on CPET outcomes. Medical researchers and doctors make those predictions on a daily bases by looking at the results of the endurance tests. They learned by many years of experience which measures are useful for predicting if a patient has a high risk of a clinical event. However, our feature selection algorithms look at all the available data and select features that are useful for prediction, without being influenced by years experience. By using this technique, we were able to find new and unexplored predictive measures.
The feature selection algorithms demonstrated that information in the recovery phase of a CPET test could improve the prediction of future clinical events (hospitalization, surgery or otherwise). Normally, (pediatric) cardiologists mainly look at the exercise phase of CPET’s, as opposed to the recovery phase, when predicting whether a patient needs attention. Further research is carried out to understand whether this information is indeed useful for doctors in their future predictions.
Moreover, our model confirmed the doctors’ suspicions that predicting future clinical events is difficult for children with a congenital heart disease. With adults it is easier to determine the current health of a patient and predict the possibility of such an event. One of the reasons for this is that it is harder to push children to their physical limits in a CPET. This leads to measures that are less accurate, which leads to difficulty in predictions, both for doctors and for our model.
Automating data interpretation
Figure 3. Dashboard to automate the interpretation of CPET’s. (dummy data)
A lot of work in research consists of interpreting datasets. For Stichting Hartekind researchers need to determine the anaerobic threshold for around 1.300 CPET’s. Firstly, this work is time consuming. It involves opening individual data files, plotting graphs, analysing their outcomes and exporting the results. Secondly, measuring the anaerobic threshold from CPET data is difficult, especially for CPET of children. These datasets are often quite noisy and can be hard to analyse visually. We helped to automate this work and helped with detecting the anaerobic threshold automatically. We built a model that is able to detect the anaerobic threshold consistently. Moreover, we created a dashboard to streamline the analysis process for doctors. This will save researchers and doctors valuable time. Furthermore, we will investigate whether our data driven model improves the accuracy of determining the anaerobic threshold. The initial findings look promising.
We really enjoyed this pro-bono project for Stichting Hartekind and look back on a successful Collective Week in Germany. Looking forward, we hope that data science can contribute more to research in general as it can help in different data related problems and gives researchers a boost in their work.
Would you like to know more?
Get in touch with Jelmer Quist at jquist@adc-consulting.com or check our contactpage.
What stage is your organisation in on its data-driven journey?
Discover your data maturity stage. Take our Data Maturity Assessment to find out and gain valuable insights into your organisation’s data practices.