How to deal with ineffective detection of financial crime transactions and money laundering? In this insight experts explain our end-to-end approach to transaction monitoring and anti-money laundering.
Key Takeaways
- Financial institutions invest heavily in resources and techniques to improve the detection of money laundering and financial crime transactions
- The task at hand remains challenging, even though more sophisticated models are available to detect anomalies in transaction patterns
- Financial institutions still suffer from a high number of false positives. If so, it is important to revise the overall transaction monitoring framework.
- Looking solely at inefficient models or business rules will at best address the symptoms. It will not improve the efficiency and effectiveness of the entire framework
- Build the transaction monitoring framework as an end-to-end risk management framework
What is the Challenge in Detecting Money Laundering and Financial Crime?
Financial Institutions (banks, payment service providers, insurers, etc.) still face a major challenge. Namely, large amounts of false positives in the detection of money laundering and financial crime in financial transactions. There are several reasons for this:
- The total volume of financial transactions is still growing
- Criminals come up with increasingly sophisticated techniques to launder money and/or finance criminal activities
- Only a few examples of money laundering and terrorism are available, especially for smaller financial institutions. This makes it difficult to design specific rules or even train sophisticated Machine Learning (ML) models
- Departments involved in detecting money laundering/financial crime often work in silos. As a result of which different steps in the monitoring framework are insufficiently aligned and connected. This results in teams trying to address symptoms rather than the root causes of inefficiency
- A model testing and change framework for detection business rules and models has not been thoroughly developed or implemented
Focusing too much on reducing false positives in the transaction monitoring systems can increase the risk of false negatives. False negatives mean not detecting money laundering and criminal activities. Consequently, this can have severe regulatory repercussions. Therefore, improving the transaction monitoring system has to be done with care. It is often necessary to take a few steps back and look at all the steps in the transaction monitoring framework. First of all, are all subprocesses in the transaction monitoring framework still connected in a sensible way? And second of all, are all teams involved in the transaction monitoring framework still working together in an efficient and effective way?
Transaction Monitoring Framework as an End-to-end Risk Management Framework
It is tempting to fix only specific components in the framework, for example, when the current transaction monitoring framework shows inefficiencies in terms of large amounts of false positives. Or when, even worse, money laundering and/or financial crime transactions go undetected. An example of a quick fix is a model or business rule that produce over 99% false positives. A natural reaction when this happens is to improve the business rule. Or to start building more sophisticated models. That have the potential to detect more complex patterns and anomalies in transaction behaviour. So, making more complex models can have its merits. But only do this after reviewing the overall transaction monitoring framework to see if all pieces are still connected.
A “good-old-fashioned” end-to-end risk management framework is a good approach to realign and improve the steps in the transaction monitoring framework. In Figure 1, the steps in this framework are envisioned as a feedback loop. In this way the framework is regularly improved and updated, rather than as a one-time fix.
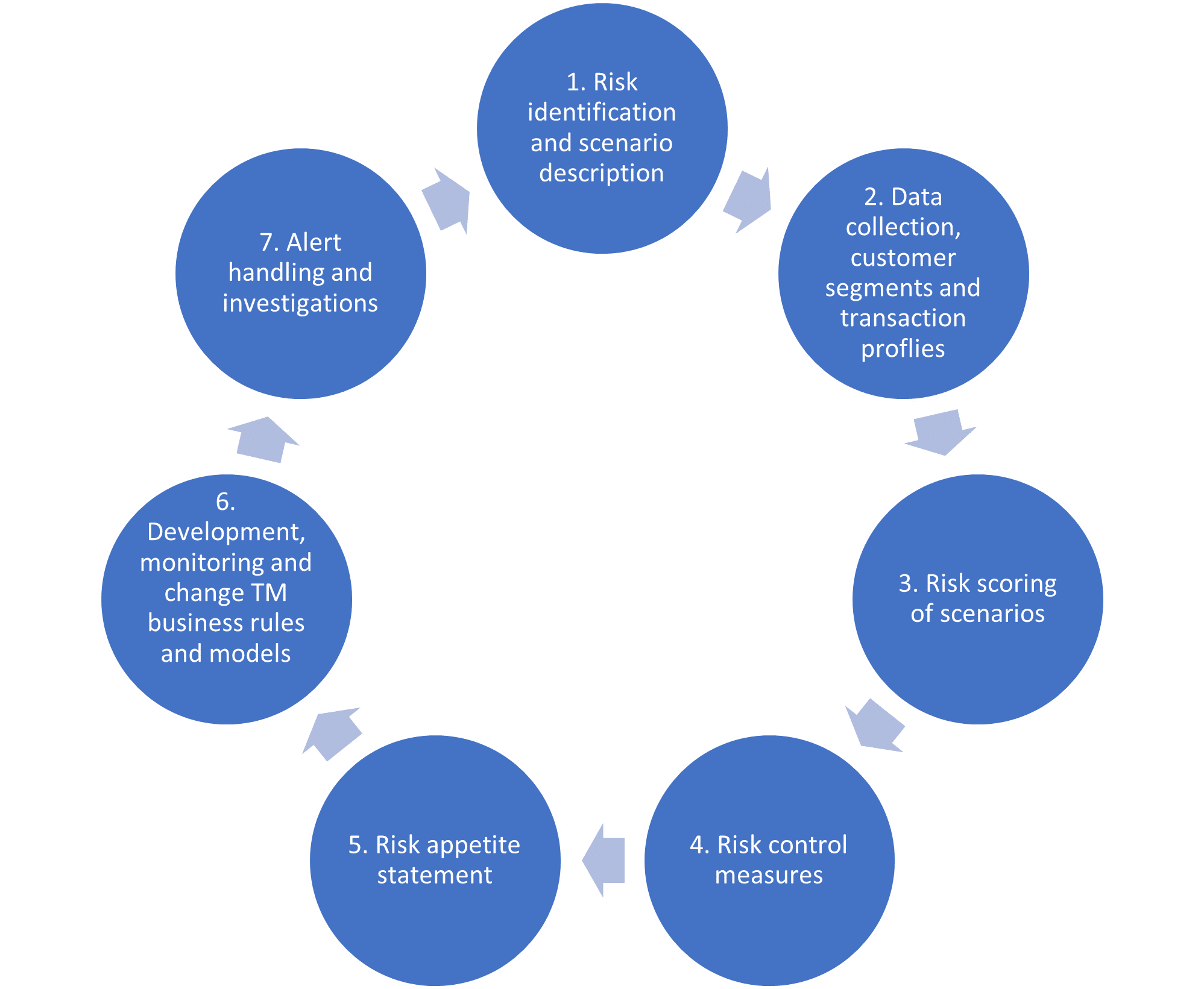
- Risk identification by describing detailed risk scenarios
The risk identification step is part of the Systematic Integrity Risk Assessment (SIRA). Usually, financial institutions organise workshops involving both 1st line operations and 2nd line Compliance and Operational Risk Management (CORM). This is to identify Money Laundering/Financial Crime (ML/FC) risks relevant to the business model of the company. In the workshops, a number of sources describe risk scenarios, among others:
- A customer’s risk profile as identified in the Customer Due Diligence (CDD) process
- Suspicious transactions identified in the past; these activities may have led to escalation to the compliance team or even to reporting to the FIU
- New ways of ML/FC identified by central banks, regulators, industry associations such as the FAFT, and the FIUs/Egmont Group
- Academic research
- News feeds on specific ML/FC cases
A scenario can be specifically related to a customer, or a customer segment as determined in Step 2. A common challenge in this step is that the descriptions of scenarios are too broad. This makes it difficult to produce targeted risk control measures in Step 5. Therefore, it is essential to add relevant details and granularity to each risk scenario.
2. Data collection, customer segmentation and expected transaction profiles
In this step, identification of suspicious activities takes place by using data to segment customers and transactions. The activities are described in the scenarios from Step 1. Use of KYC and transaction data segments customers and/or transactions based on risk factors. For example, the type of customer, customer segment (gambling, gaming, charities, etc), high-risk countries, payment method (cash, credit cards, bitcoins, gift cards, etc) and the nature of the transactions (customer to customer, merchant to customer, chargebacks and refunds, etc). Transaction data can also be used to better understand the expected transaction behaviour of customers or customer segments. By defining these segments, it is possible to construct specific rules and/or models for specific business types and industries. This results in a more tailor-made transaction monitoring system instead of a one-size-fits-all approach.
3. Risk scoring of scenarios
The risk score is based on a predetermined scoring method used in the overall SIRA. In the SIRA workshops, the CORM team evaluates how an occurring scenario of Step 1 affects the FI. As a result of these evaluations the production of a heatmap matrix takes place. This shows the probability and impact of the gross risk (risk before any control mechanisms).
Finally, it is important that the score is regularly reviewed. Using information from the alert handling system/feedback form analysts, new information for the KYC process and other relevant information from the transaction monitoring framework.
4. Risk control measures
Following Step 3, the CORM team determines the level of controls for the different scenarios. Examples of controls are:
- A business rule: usually a one-dimensional rule to detect deviations from the expected transaction profile
- More complex statistical models to determine anomalies in transaction behaviour patterns (the complexity ranges from statistical regression and clustering techniques to deep-learning machine learning models with multiple layers)
- A (change in) policies or procedures with regard to, for example, acceptance and exit procedure of customers/customer segments
- Other tooling, for example tools to identify networks of criminals conspiring, to carry out ML and/or FC.
5. Risk appetite statement
The CORM team is responsible for establishing a risk appetite statement. Senior management of the financial institution approves the statement. For each of the scenarios identified in Step 1, the CORM team determines the net risk after applying the control measures in Step 4. Additional measures are necessary when the net risk exceeds the risk appetite.
6. Development, monitoring and change management of transaction monitoring business rules and models
The development of transaction monitoring business is closely linked to the scenarios described in Step 1. How can the ML/FC behaviour in the scenario be detected by the business rules? The development of more advanced models takes place where business rules fail to detect suspicious activity. The models can detect complex patterns in transactions and transaction behaviour. Moreover, the more advanced models rely on statistical techniques to detect correlations and clusters of suspicious activities. Training these models can be challenging when the number of documented true positives is small. Mainly banks are already working together to share data to tackle this challenge.
Model Governance of transaction monitoring Business Rules and Models
We advise that the development and maintenance of business rules and models follow the same governance as (financial institutions are familiar with) in financial risk modelling:
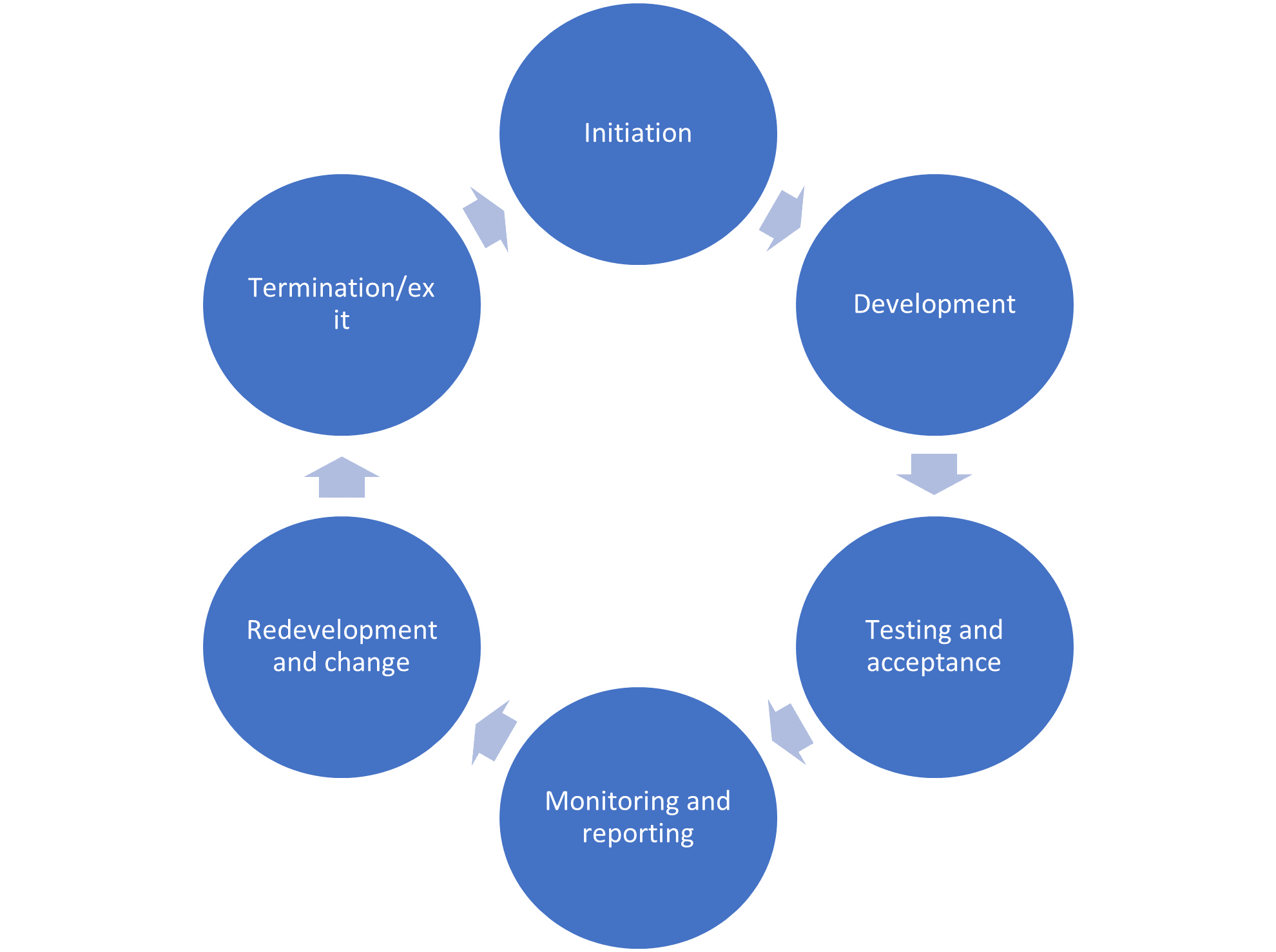
- Initiation: Building a business case for a new business rule or model takes place. What scenario or behavioural patterns does the rule or model attempt to capture? Is this a scenario from the SIRA process that has not been captured before? What is the expected effectiveness?
- Development: Developing a new business rule or model takes place when the business case is positive. It is important that a business rule or model[MA|A1] can be traced back to a scenario in the risk identification step
- Testing and acceptance: Testing of the new business rule or model takes place. Is it capturing suspicious behaviour is a historic training set (potentially augmented with synthetic cases of ML/FC)? The business rule or model goes into production when it meets the functional and user acceptance criteria. It may be contemplated to run more complex models in a shadow environment. This is to test effectiveness before going into production mode
- Monitoring and reporting: The next step is monitoring the performance of business rules or models and reporting to senior management. The monitoring step can have a feedback loop into the risk identification step of the SIRA. Namely, are unusual patterns detected by models captured in specific SIRA scenarios?
- Redevelopment and change: Redevelopment takes place when business rules and models underperform. For a business rule this can also mean that a threshold can change
- Termination/exit: The termination of business rules and models with poor performance takes place and newly developed once replace them.
7. Alert handling and investigations
The Alert Handling team handles alerts from business rules and models. Suspicious activities are escalated to compliance, who may decide to file a report to the FIU. It is important that analyses of the alert handling team feed back to the scenario descriptions in Step 1 and in the development of business rules and models in Step 6. It helps when categorisation and documentations of the analysis of suspicious takes place per, for example, risk factor. In this way it is easier to improve the SIRA scenarios. Also, the redesign of business rules/models is easier as part of this feedback loop.
Deviations from the expected transaction behaviour as identified in Step 2 should also feed back to the periodic CDD process.
Business Case to Improve the transaction monitoring Framework
Revisiting the entire transaction monitoring framework can be a time consuming task. Before starting with a comprehensive review, some key question can be asked. This is to indicate whether the business case for investing in an improvement is strong:
- Are the scenarios in the risk identification granular enough to build effective business rules and models?
- Do all components of the transaction monitoring framework connect in a logical way (see Figure 1)?
- Do all relevant teams align in the end-to-end transaction monitoring process?
- Are current business rules and models effective in detecting ML/FC?
If two or more answers to the above questions are negative, a more thorough review can be beneficial. We expect to achieve a 25% improvement in both the reduction of false positives and increased operational efficiency of the alert handling team. Investing the time saved is valuable. In, among other things, deeper manual analyses (including feedback in the risk identification/scenario setting process) and the preparation of high-quality Suspicious Activity Reports.
How can Amsterdam Data Collective help?
In summary, Amsterdam Data Collective can help in the following ways:
- Conducting a maturity assessment of your current transaction monitoring and SIRA framework
- Assess and improve your SIRA money laundering and financial crime scenarios
- Design methodology for customer segmentation
- Develop a business rule and model test and change process
- Test effectiveness of current rules and models
- Develop new business rules/models
Feel free to reach out to us for more information, please contact Scott Bush at sbush@adc-consulting.com or check our contact page.
What stage is your organisation in on its data-driven journey?
Discover your data maturity stage. Take our Data Maturity Assessment to find out and gain valuable insights into your organisation’s data practices.